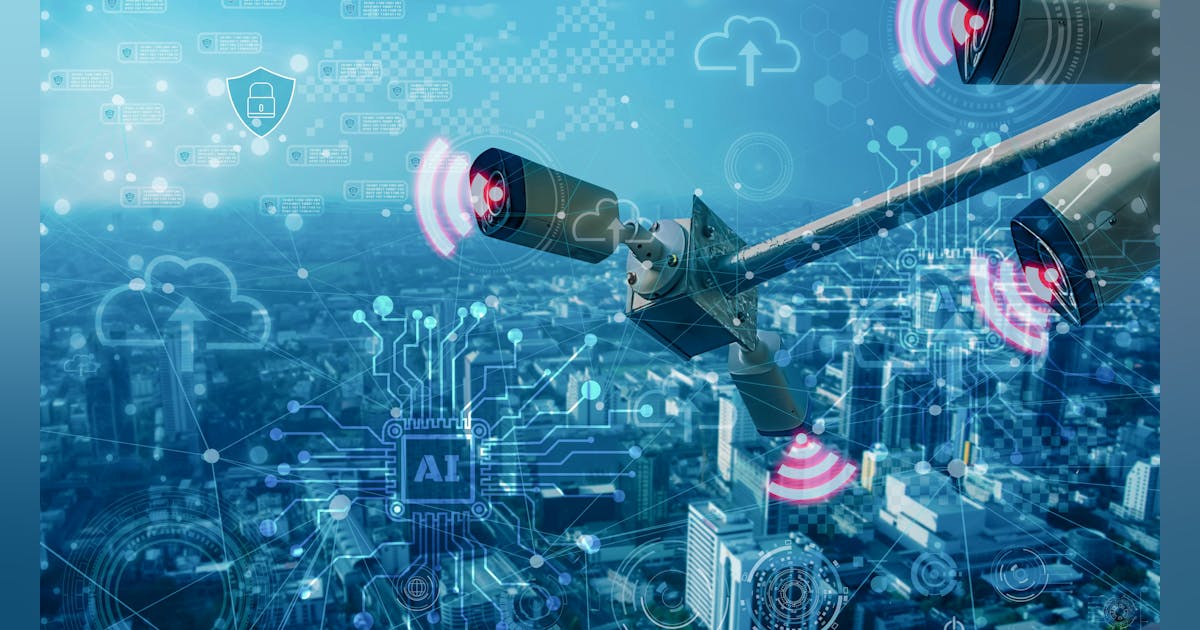
Technology Roundtable Sponsored by Hanwha Vision America
The increasing use of Artificial Intelligence (AI) across all market segments combined with higher-quality video and a wider embrace of data and analytics are creating “smarter” solutions that can deliver more accurate detection and search results, reduce system bandwidth, and increase operational efficiency, among many other benefits.
Aaron Saks, the Senior Technical Marketing and Training Manager and Ramy Ayad, a Senior Director of Product Management from Hanwha Vision America discuss a range of business and technology issues changing how security and surveillance systems are designed and deployed with Security Technology Executive magazine editors (STE).
STE: What does AI mean to video surveillance now and in the future?
Saks: The continued integration of Artificial intelligence (AI) and Machine Learning in security and surveillance systems has enabled smart video analytics, automated threat detection, and predictive analysis.
The recent trend for AI has been allowing analytics to provide meaningful alerts by eliminating false positives from trees, shadows, and other non-relevant movements. AI enables quick forensic searching by object type and attributes, such as vehicle type or color. Moving forward, AI will allow for further customization of user-specified objects to alert on and automatic optimization of a camera’s settings, such as bitrate, shutter speed, and many others, based on the scene and its content. This allows the camera to become “smarter.”
Combined with a trend of security professionals taking more control of how they manage their surveillance systems, AI-based compression and noise reduction technologies are growing in use, mainly because they give professionals more flexibility and choice. For example, users can decide if they want to reduce bandwidth or even manipulate the compression according to only the objects that are important to them during a specific forensic search.
The role of data and analytics will continue to expand significantly in 2024 and beyond, as customers combine edge computing and AI to complement and enhance data collection and analytics.
The use of Edge AI, especially with analytics based on deep learning algorithms, will be a key element in a range of smart network surveillance applications. These include object detection and classification, and the collection of attributes in the form of metadata – all while reducing latency and system bandwidth burdens and enabling real-time data gathering and situational monitoring.
Ayad: The most obvious key benefits of AI are false alarm reduction and forensic search effectiveness. The more we improve and add more attributes to the detections, the faster the forensic search and the more accurate the alarms. We also use AI to enhance the bandwidth reduction algorithm as we now focus on AI detection for a much better and more efficient bandwidth reduction. In the past, we were focused on pixel changes where any movement, like rain, snow, or video noise could cause video bandwidth to increase. Now, these pixel changes are ignored. AI is also effective in reducing video noise and motion blur.
AI models can be trained to detect anything if they have a sufficient training dataset. Generative AI is being used when collecting a dataset is too difficult or might include personal data. Now, generating a realistic yet graphical image with multiple variations like low light, snow, rain, etc. is much easier, and we believe in the future that will be the case when training AI models.
For example, in license plate recognition, there are multiple variations like language, format, and specialty license plates, and getting a real image of every single variation is almost impossible. Rather, it would be ideal if we were able to generate a graphical yet realistic license plate image with any variation. Now, we have a smarter AI model that can detect these variations without the need to collect datasets from each country and state.
STE: How does interoperability with other systems make video more applicable in multiple settings?
Saks: Interoperability is a driving factor, just like we saw with ONVIF in the past 10 years. Previously, direct driver integration was required to achieve full performance. As more systems become compatible with the latest ONVIF standards, these important feature sets will become enabled across the board, ensuring different platforms can fully use these key features. An example is with ONVIF profile M, allowing a VMS or central station to view and search for object attributes. If a VMS has the right integration, but another critical software package isn’t compatible, users are relegated to doing tasks the old-fashioned way. This allows them to use best-in-breed software that suits their needs, as opposed to being restricted or pigeonholed into what is compatible.
STE: What does AI (Artificial Intelligence) mean to video surveillance now and in the future?
Ayad: The most obvious key benefits of AI are false alarm reduction and forensic search effectiveness. The more we improve and add more attributes to the detections, the faster the forensic search and the more accurate the alarms. We also use AI to enhance the bandwidth reduction algorithm as we now focus on AI detection for a much better and more efficient bandwidth reduction. In the past, we were focused on pixel changes where any movement, like rain, snow, or video noise could cause video bandwidth to increase. Now, these pixel changes are ignored. AI is also effective in reducing video noise and motion blur.
AI models can be trained to detect anything if they have a sufficient training dataset. Generative AI is being used when collecting a dataset is too difficult or might include personal data. Now, generating a realistic yet graphical image with multiple variations like low light, snow, rain, etc. is much easier, and we believe in the future that will be the case when training AI models.
For example, in license plate recognition, there are multiple variations like language, format, and specialty license plates, and getting a real image of every single variation is almost impossible. Rather, it would be ideal if we were able to generate a graphical yet realistic license plate image with any variation. Now, we have a smarter AI model that can detect these variations without the need to collect datasets from each country and state.
STE: What are the top industries using video surveillance?
Saks: All vertical industries have adopted video surveillance; however, we are now seeing a shift away from lowest-cost bare-bones systems to system integrators developing solutions that truly take advantage of these cutting-edge technologies. We are seeing schools deploying more cameras with AI and intelligent factory solutions can use AI to alert when abnormal activities occur.
In many markets such as cannabis or banking, there are regulations stating that operations must have both on-site and off-site back-up storage. Cannabis has been a huge growth opportunity as legislation in many areas requires 24×7 video from seed to market. Finally, banking and retail are maturing, moving away from limited camera counts due to budget concerns to embracing AI to provide meaningful data about operations, while also improving loss prevention activities.
In a casino, AI-powered analytics can boost the already-strong security capabilities of a camera by helping casino operators understand guest behavior patterns, determine the busiest, and slower, times of the day, which games are more regularly visited, and more. Managers can decide where to place staff for optimal coverage depending on traffic.
This diversity across sectors shows just how far-reaching AI has become, not only for surveillance but for every market segment.
Ayad: We’re seeing increasing and diverse uses of AI in many market segments.
For example, in schools, combining AI with digital imaging surveillance technology and onboard audio and video analytics can help school administrators with the monitoring of hallways, classrooms, and exterior parking lots. For example, knowing which doors visitors can use to access and exit a building is important when deciding where to place cameras. These analytics deliver actionable data that can drive intelligent monitoring for education facilities.
The use of AI is spreading across the healthcare industry to enhance patient care, improve operational efficiency and improve patient care. From a security and surveillance perspective, hospitals are complementing their cameras’ security monitoring performance with enhanced data-gathering capabilities combining intelligent audio/video analytics and AI.
Hospitals are using Artificial Intelligence (AI) combined with video analytics to help manage their networks of cameras and devices, shifting their security and surveillance approach from reactive to proactive. The result is targeted object detection and classification, which can save time for hospital security teams by speeding forensic searches.
In retail environments, new AI technology has added the power to do people-counting, body temperature detection, object detection, license plate recognition, behavioral observations, and any number of actionable applications. These types of video solutions address the industry’s need for scalable and cost-effective surveillance solutions that can help organizations monitor their stores and detect suspicious activity, preventing theft.
STE: How does interoperability with other systems make video more applicable in multiple settings?
Ayad: We have seen in recent years a heightened awareness of the need for high-quality, reliable, and stable video. Security professionals realize their devices need to do more than just monitor and protect people or a facility. These products are becoming part of total business solutions, being used to deliver data-driven business insights and drive enhanced operational efficiencies across an entire organization. This places a greater emphasis on the development of high-performance optical and image-processing technologies, and we are seeing these designs come to reality in the form of cameras built for every security and surveillance application.
STE: How is AI enabling other surveillance applications, such as remote system monitoring, cloud-based services, analytics, and data gathering?
Saks: AI allows cloud and remote monitoring solutions to effectively manage bandwidth by only alerting when an important event is triggered, such as an unauthorized person in an area, loitering, etc. Previously, cloud recording was limited to continuous recording and processing motion detection in the cloud, whereas now edge AI processing can be coupled with a cloud solution.
Many remote monitoring solutions would use simple SMTP e-mail notifications and short video clips. Now, detailed metadata can be transmitted allowing an operator to quickly see relevant information, while only receiving notifications on pertinent items. When an incident occurs, locating a person of interest can take a matter of minutes instead of having to spend hours sifting through hundreds of camera streams.
Ayad: Customers are looking for solutions that match the new ways of working they have had to adopt in recent years. This will accelerate the continued acceptance of constantly evolving technologies including AI, edge recording and cloud platforms. Video performance and enhanced image quality will be more important than ever to deliver precise detection of people, objects, and vehicles; robust search capabilities; and elevated data analysis capabilities. We see AI going in many directions like audio detection, anomaly detection, custom object detection, and many others.
Meet the Experts:
link